vLLM vs. TensorRT-LLM: Which Inference Library is Best for Your LLM Needs?
.png)
Introduction
This blog explores two inference libraries: vLLM and TensorRT-LLM. Both are designed to optimize the deployment and execution of LLMs, focusing on speed and efficiency.
vLLM, developed at UC Berkeley, introduces PagedAttention and Continuous Batching to improve inference speed and memory usage. It supports distributed inference across multiple GPUs.
TensorRT-LLM is an open-source framework from NVIDIA for optimizing and deploying large language models on NVIDIA GPUs. It leverages TensorRT for inference acceleration, supports popular LLM architectures, and offers features like quantization and distributed inference.
Comparison Analysis
Performance Metrics
vLLM and TensorRT-LLM are popular solutions for deploying large language models (LLMs), renowned for their efficiency and performance. We will compare them based latency, throughput, and time to first token (TTFT):

Features
Both vLLM and TensorRT-LLM offer robust capabilities for serving large language models efficiently. Below is a detailed comparison of their features.

Ease of Use
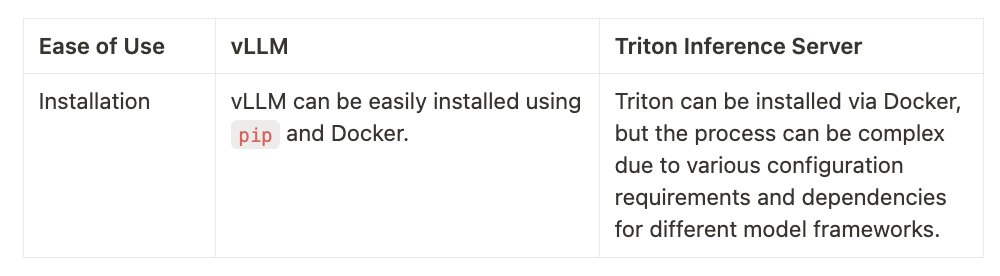
Scalability
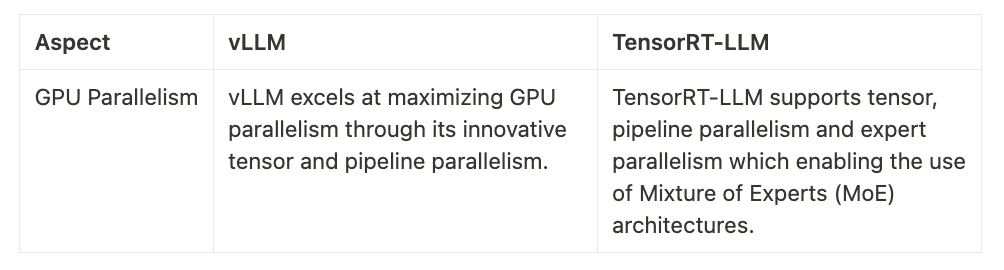
Integration
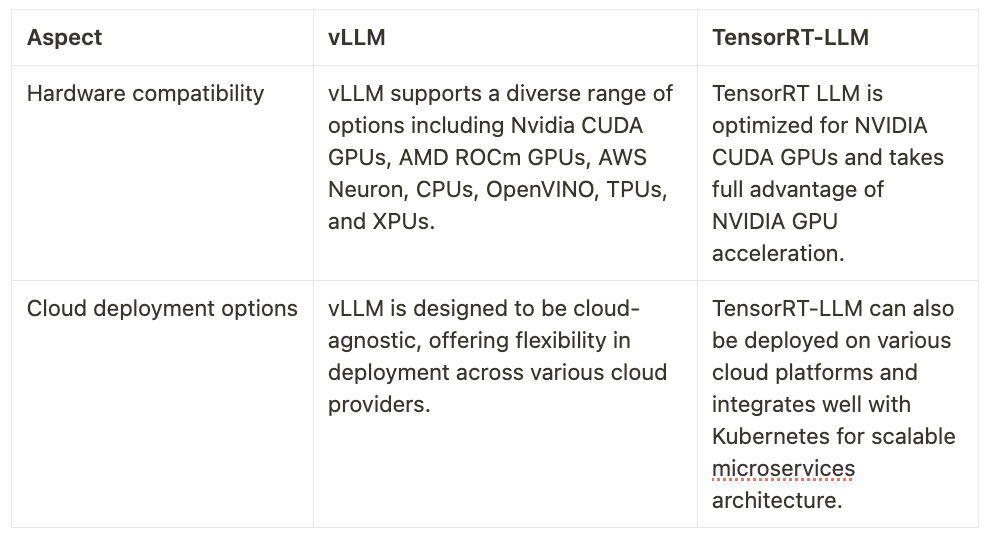
Conclusion
Both vLLM and TensorRT-LLM offer powerful solutions for serving large language models (LLMs), each with unique strengths tailored to different deployment needs. vLLM stands out with its innovative features like PagedAttention and Continuous Batching, which significantly enhance inference speed and memory efficiency. Its cloud-agnostic design and support for a wide range of hardware platforms make it a versatile choice for organizations seeking to optimize LLM deployment.On the other hand, TensorRT-LLM excels with its deep integration with NVIDIA hardware, for serving NVIDIA's specialized models and offering quantization techniques that can leverage the full capabilities of NVIDIA GPUs. This makes TensorRT-LLM particularly attractive for deployments that are centered around NVIDIA ecosystems and require intensive computational tasks to be executed with high efficiency.
Ultimately, the choice between vLLM and TensorRT-LLM will depend on specific project requirements, ease of use, and existing infrastructure. Both libraries are under continuous development, rapidly adapting to meet user demands and broadening their range of capabilities.
Resources
- https://github.com/NVIDIA/TensorRT-LLM/
- https://arxiv.org/html/2403.02310v1
- https://www.databricks.com/blog/llm-inference-performance-engineering-best-practices
- https://blog.vllm.ai/2024/09/05/perf-update.html
- https://docs.nvidia.com/tensorrt-llm/index.html