vLLM vs. DeepSpeed-MII: Choosing the Right Tool for Efficient LLM Inference
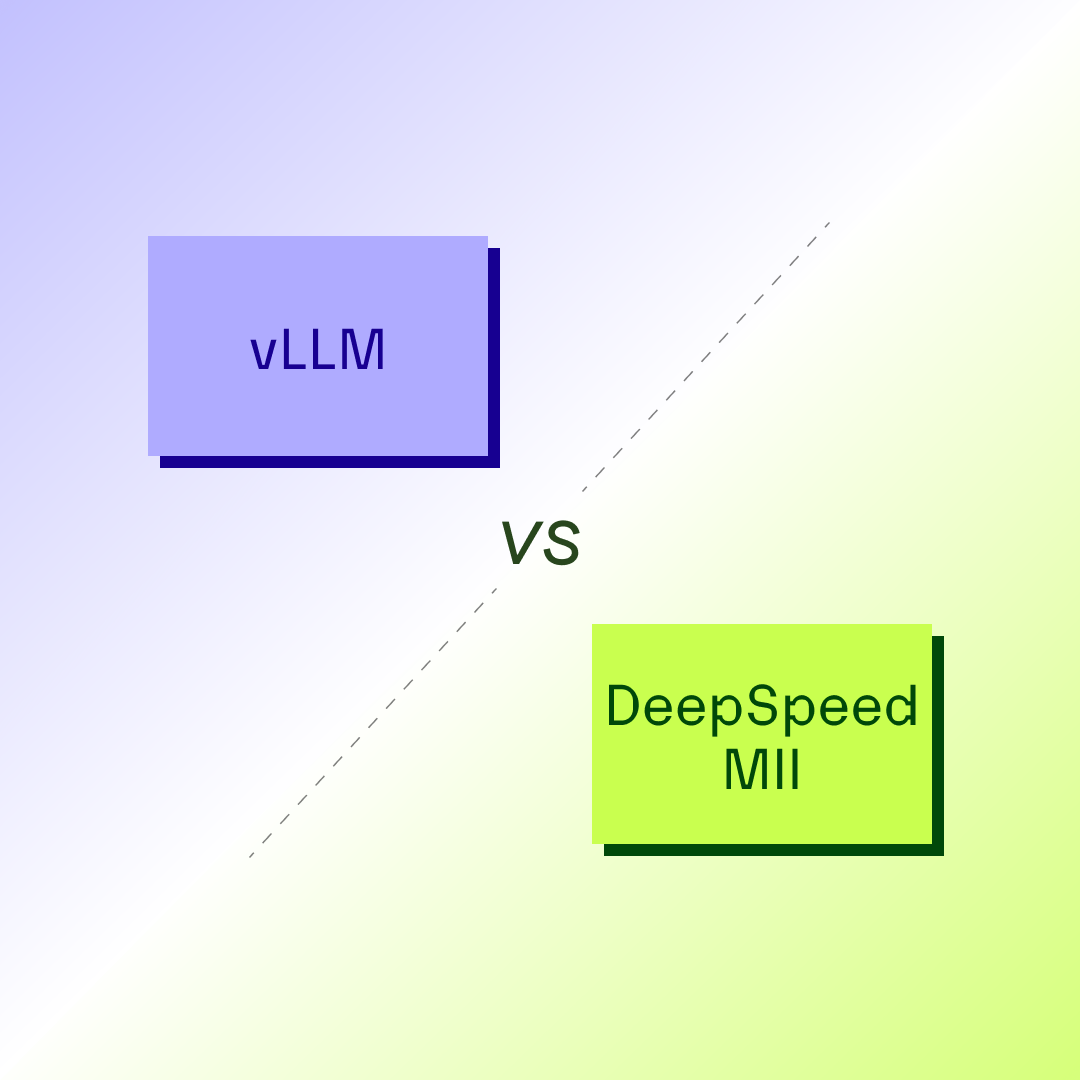
Introduction
This blog explores two inference libraries: vLLM and DeepSpeed-MII. Both are designed to optimize the deployment and execution of LLMs, focusing on speed and efficiency.
vLLM, developed at UC Berkeley, introduces PagedAttention and Continuous Batching to improve inference speed and memory usage. It supports distributed inference across multiple GPUs.
DeepSpeed-MII, an open-source Python library created by the DeepSpeed team to make powerful model inference accessible to all. It emphasizes high-throughput, low latency, and cost efficiency.
Performance Metrics
DeepSpeed-MII and vLLM are popular solutions for deploying large language models (LLMs), renowned for their efficiency and performance. We will compare them based latency, throughput, and time to first token (TTFT):
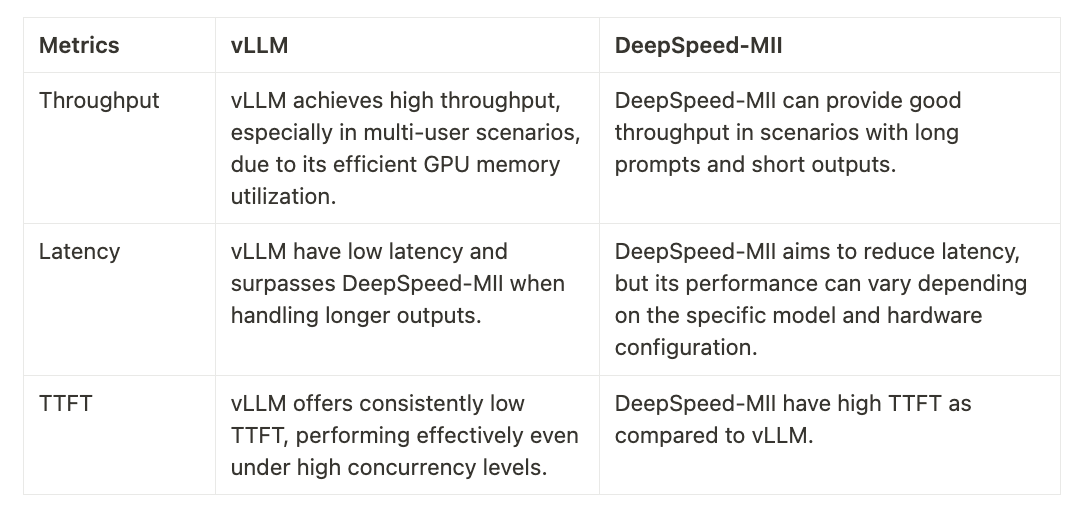
Features
Both vLLM and DeepSpeed-MII offer robust capabilities for serving large language models efficiently. Below is a detailed comparison of their features.
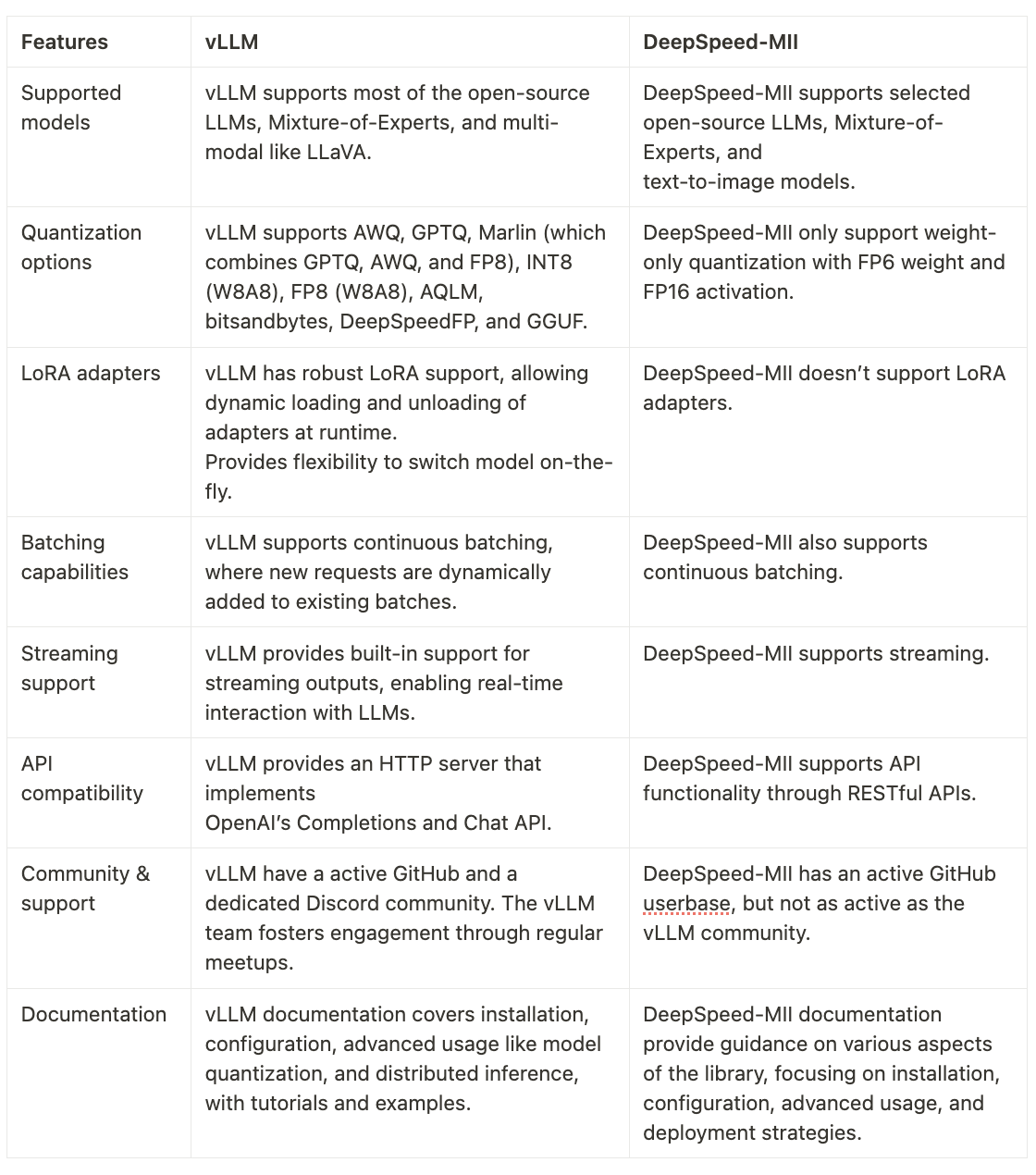
Ease of Use

Scalability

Integration
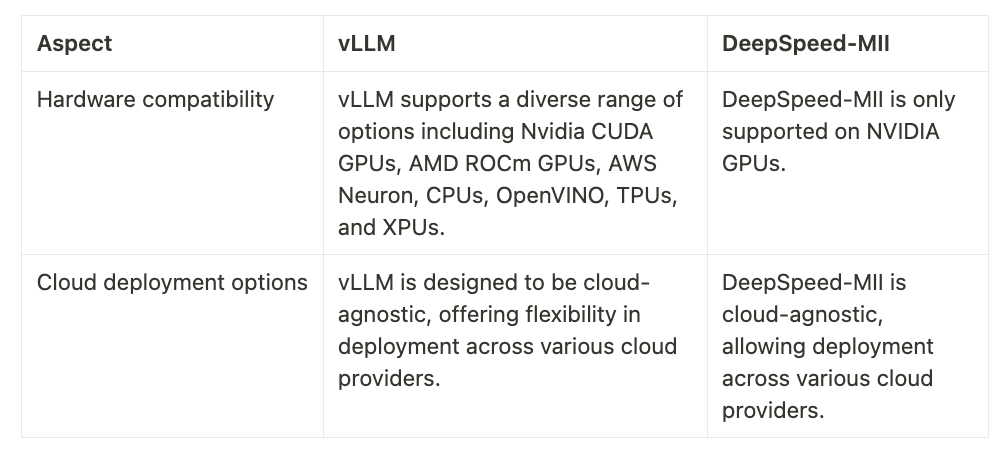
Conclusion
Both vLLM and DeepSpeed-MII offer powerful solutions for serving large language models (LLMs), each with unique strengths tailored to different deployment needs. vLLM stands out with its innovative features like PagedAttention and Continuous Batching, which significantly enhance inference speed and memory efficiency. Its cloud-agnostic design and support for a wide range of hardware platforms make it a versatile choice for organizations seeking to optimize LLM deployment.
On the other hand, DeepSpeed-MII shines in scenarios with long prompts and short outputs. It offers strong support for weight-only quantization, which can be valuable for certain environments. Although its community support and documentation are not as expansive as vLLM, it still remains a strong contender due to its proven efficiency in serving LLMs.
Ultimately, the choice between vLLM and DeepSpeed-MII will depend on specific project requirements, including performance metrics, ease of use, and existing infrastructure. As the demand for efficient LLM serving continues to grow, both libraries are poised to play critical roles in advancing AI applications across various industries.
Resources
- https://arxiv.org/html/2401.08671v1
- https://github.com/microsoft/DeepSpeed-MII
- https://blog.vllm.ai/2023/11/14/notes-vllm-vs-deepspeed.html
- https://www.microsoft.com/en-us/research/project/deepspeed/deepspeed-mii/
- https://deepspeed-mii.readthedocs.io/en/latest/