Distilling Large Language Models
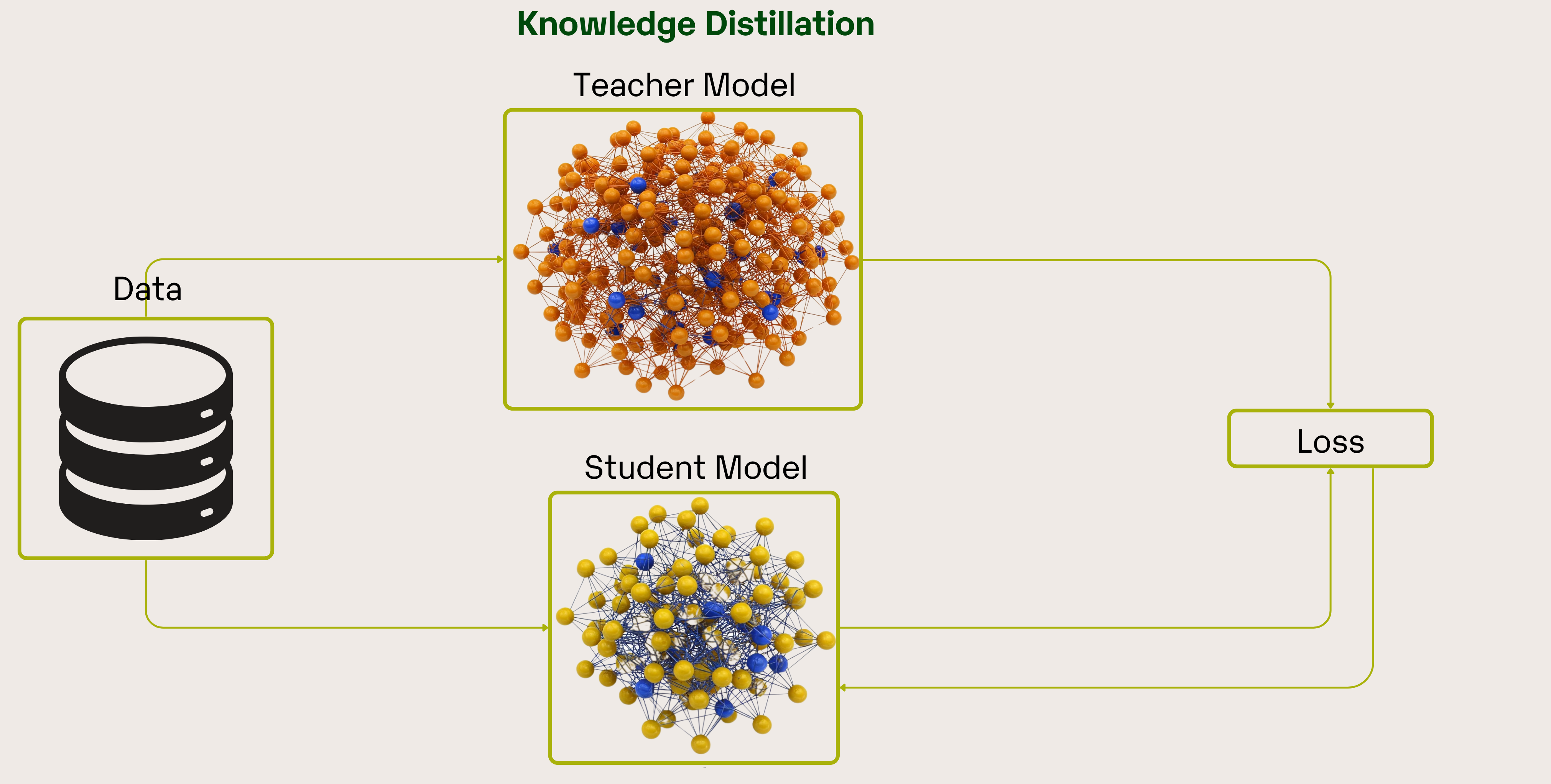
Introduction
Large Language Models (LLMs) such as DeepSeek-R1, GPT-4.5 have completely revolutionized natural language processing by demonstrating unprecedented capabilities in understanding and generating human-like text, as detailed in an overview of neural networks. These models leverage deep neural network architectures primarily transformers to capture complex patterns and long-range dependencies in language.
Despite their groundbreaking performance, LLMs pose significant challenges when it comes to real-world deployment. These factors not only drive up operational costs but also hinder real-time applications especially on edge devices or in resource-constrained environments.
Knowledge distillation offers a promising solution by transferring the “knowledge” of a large teacher model into a smaller student model. This process is designed to retain most of the predictive power and nuanced decision-making capabilities of the teacher model while significantly reducing its size and computational demands. As a result, the distilled model can deliver near-equivalent performance with faster inference and lower resource consumption, making it ideal for deployment in production environments.
Understanding Knowledge Distillation
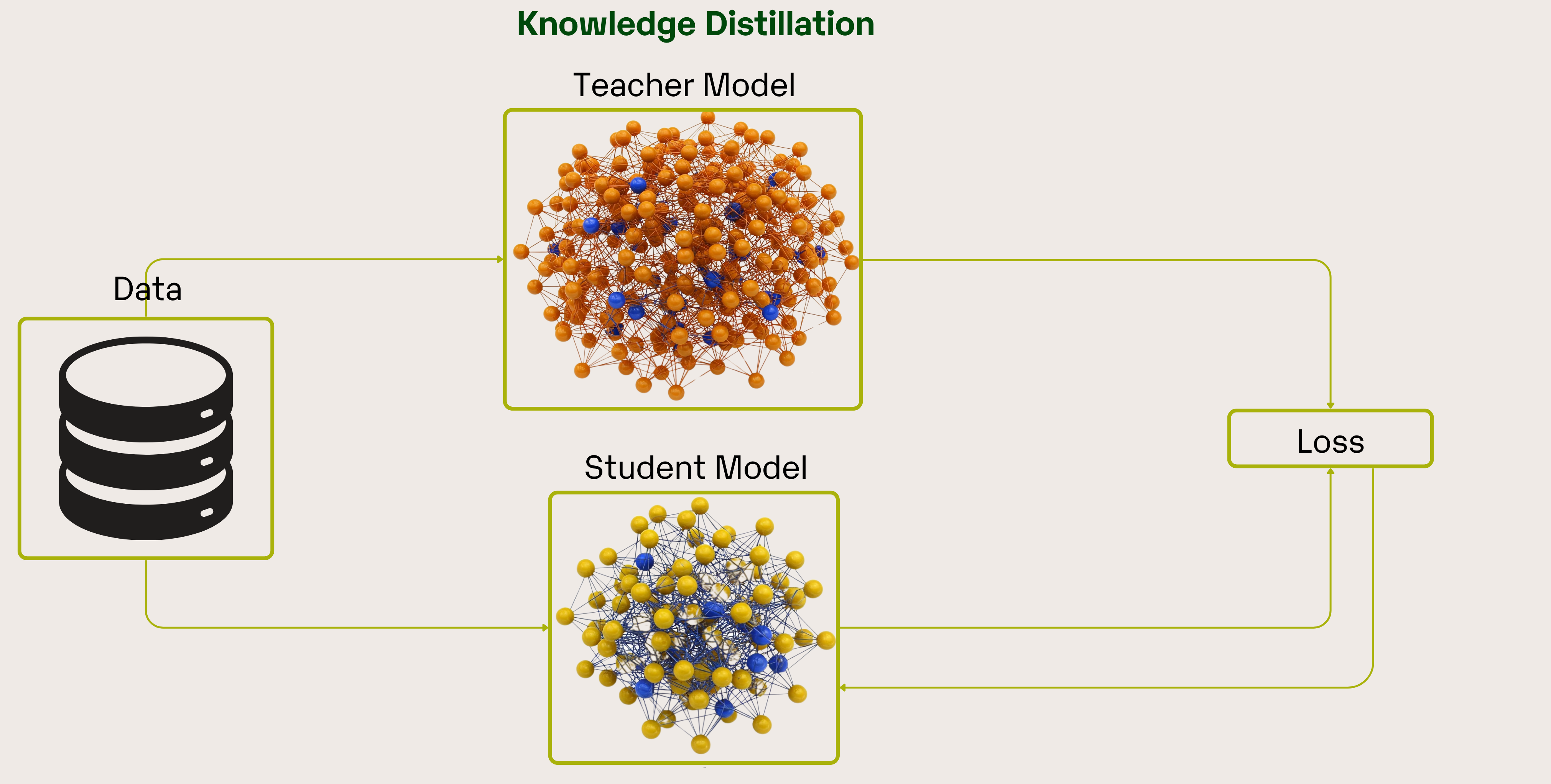
The concept of knowledge distillation was popularized by Hinton, Vinyals, and Dean in their influential paper “Distilling the Knowledge in a Neural Network”, which builds on the principles of neural networks and deep learning.
Knowledge distillation uses a smaller student model, which is trained on the output of the teacher’s model, which is often referred to as soft targets. Soft targets provide a probability distribution over classes, reflecting not just the correct answer but also the relative confidence in alternative outcomes. This extra layer of information helps the student model learn more generalized and robust representations, enabling it to achieve competitive performance even with fewer parameters.
Mechanisms of Distillation
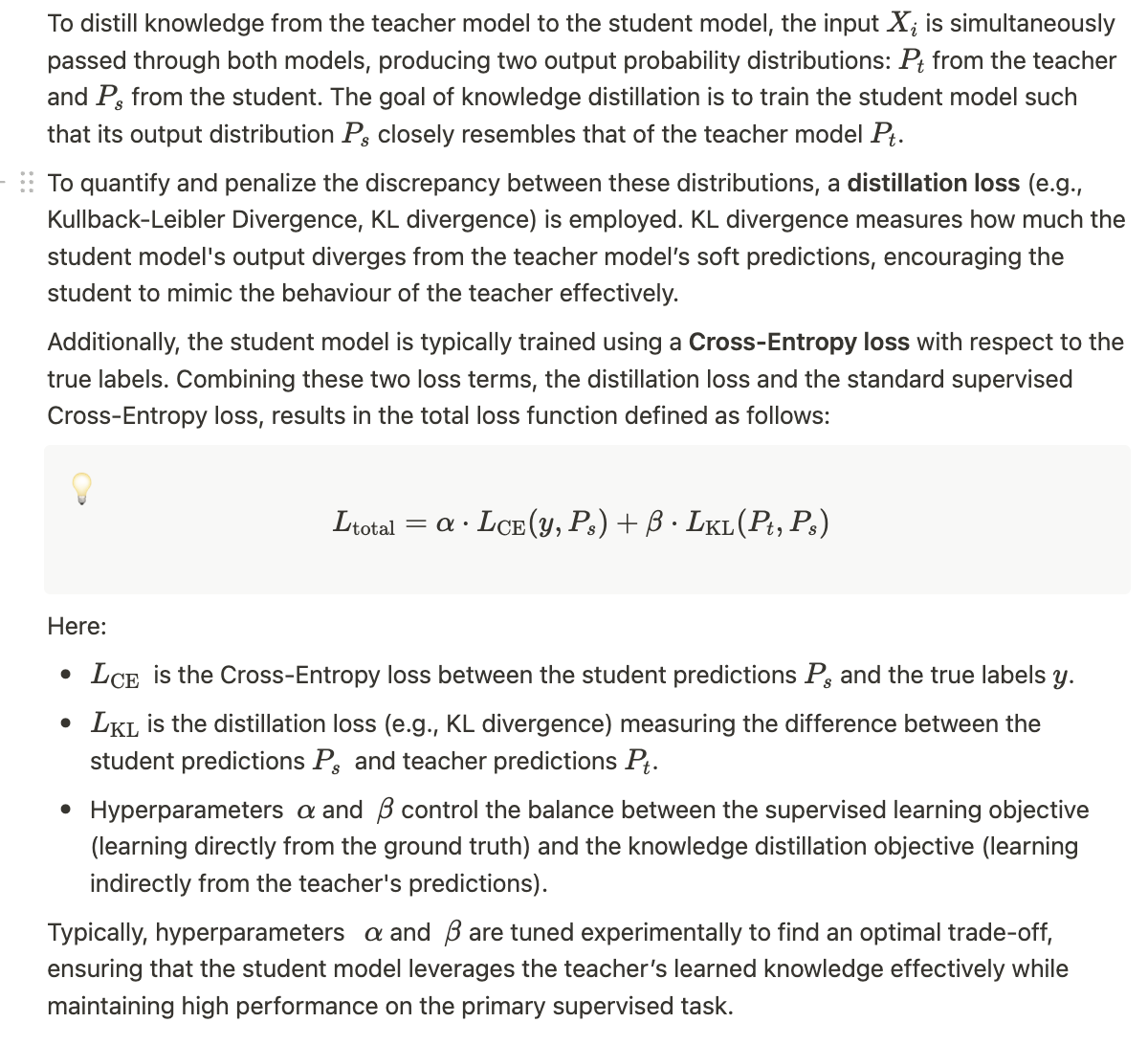
Techniques for Distilling Large Language Models
We dive into the core learning approaches used in the distillation process, including supervised fine-tuning, divergence minimization, reinforcement learning, and rank optimization as explained in Google's guide on neural networks.
1. Supervised Fine-Tuning (SFT)
Supervised fine-tuning (SFT) is one of the simplest yet most powerful distillation methods. This technique involves training a smaller student model to closely mimic the output sequences produced by a larger teacher model. By minimizing the discrepancy between student-generated outputs and teacher-provided examples, the student acquires advanced skills and capabilities present in the teacher, leading to more efficient yet capable models.
2. Divergence Minimization
Divergence minimization methods go beyond imitating the final outputs. Instead, they involve aligning the internal representations or probability distributions of the student model with those of the teacher. Techniques often employ divergence metrics like Kullback-Leibler Divergence (KLD) to measure how closely the student mirrors the teacher's internal features or token-level distributions.
This deeper level of alignment helps students capture nuanced knowledge, reasoning patterns, and decision-making processes, thereby improving cognitive abilities.
3. Reinforcement Learning (RL)
Reinforcement Learning (RL) offers an advanced method for distilling Large Language Models (LLMs) by using teacher feedback to refine student models iteratively. In this approach, a teacher model evaluates student-generated outputs, providing feedback such as preferences or corrections.
First, a reward model is trained to distinguish between more and less preferable outputs, guided by the teacher’s feedback. Then, the student model is optimized to generate outputs that maximize these rewards, aligning closely with the teacher’s preferences. This interactive cycle significantly improves the student model's capabilities, enabling it to handle complex tasks and align more closely with desired behaviors.
4. Rank Optimization
Ranking optimization offers a stable and efficient alternative to reinforcement learning (RL) for distilling preference feedback into language models. Unlike RL, which can be computationally intensive, ranking optimization directly teaches student models to produce preferred outputs, guided by explicit rankings provided by a teacher model.
In rank optimization, a teacher model first evaluates several student-generated responses, ranking them from most to least preferred. The student model is then trained to prioritize higher-ranked responses, making it directly aware of the teacher’s preference patterns. Techniques such as Preference Ranking Optimization (PRO) and Rank Responses to Align Human Feedback (RRHF) allow student models to effectively capture nuanced teacher preferences in a stable, computationally efficient manner.
Models trained through rank optimization methods, like Zephyr, have shown impressive results, achieving strong alignment with teacher preferences without the computational complexity of reinforcement learning.
Knowledge Distillation in the LLMs Era
Initially, before the widespread adoption of LLMs, knowledge distillation primarily aimed at compressing neural networks reducing computational demands and memory requirements for deployment in resource-limited settings, such as mobile or edge computing environments. Traditional approaches relied heavily on methods such as soft target training, in which smaller networks were trained to mimic the softened output distributions of the teacher model.
Due to the recent emergence of LLMs, the modern era of distillation emphasizes knowledge elicitation and targeted capability transfer. Current approaches extend beyond simple knowledge extraction, emphasizing the transfer of abstract cognitive patterns such as reasoning processes, preference alignment and value alignment. The focus is no longer limited to matching outputs between teacher and student models instead, the goal is to replicate the underlying thought processes and decision-making behaviours of the LLM teacher. Techniques such as chain-of-thought prompting exemplify this trend, encouraging student models to internalize and emulate the reasoning pathways of their teachers, thereby significantly enhancing their cognitive capabilities.
Data Augmentation in LLM-Based Distillation
Data Augmentation (DA) has evolved beyond traditional methods like paraphrasing or back-translation, which primarily increased dataset size mechanically. Now, DA strategically generates context-rich, domain-specific datasets designed explicitly to enhance knowledge distillation from powerful proprietary models to open-source alternatives.
This approach leverages carefully crafted prompts, enabling LLMs to produce datasets that expand training data not only quantitatively but also qualitatively enhance diversity, domain relevance, and skill specificity.
DA acts as a force multiplier by enabling distilled models to develop and refine their capabilities more efficiently. This integration dramatically reduces the resources needed to achieve advanced performance, leading to a shift toward a more efficient, sustainable, and accessible way to harness the power of LLMs.
Distillation Process: How to Distill an LLM
Knowledge distillation allows you to transfer the intelligence from a larger, more cumbersome teacher model to a smaller, more efficient student model.
In this example, we distill the Qwen2-7B-Instruct model into a Qwen2-1.5B-Instruct model using the DistillFlow library.
Step 1: Clone the Repository & Install Dependencies
Start by cloning the DistillFlow repository and setting up your environment. Run the following commands in your terminal:
git clone https://github.com/horus-ai-labs/DistillFlow.git
cd DistillFlow
pip3 install poetry
poetry install
These commands download the repository, install Poetry (a dependency manager for Python), and install all required packages.
Step 2: Configure the Distillation Process
Next, set up your configuration file to define the student and teacher models, datasets, and training parameters. Here’s an example configuration file:
student_model:
model_name_or_path: Qwen/Qwen2-1.5B-Instruct
flash_attn: sdpa
use_unsloth: false
output_attentions: false
enable_liger_kernel: true
deepspeed_config: './deepspeed/zero0.json'
chat_template: "{% for message in messages %}{% if loop.first and messages[0]['role'] != 'system' %}{{ '<|im_start|>system\nYou are a helpful assistant.<|im_end|>\n' }}{% endif %}{{'<|im_start|>' + message['role'] + '\n' + message['content'] + '<|im_end|>' + '\n'}}{% endfor %}{% if add_generation_prompt %}{{ '<|im_start|>assistant\n' }}{% endif %}"
teacher_model:
model_name_or_path: Qwen/Qwen2-7B-Instruct
flash_attn: sdpa
use_unsloth: false
output_attentions: false
enable_liger_kernel: true
deepspeed_config: './deepspeed/zero0.json'
# quantization_args:
# quantization_bit: 8
# quantization_method: gptq
data:
seed: 42
text_field: "text"
train_datasets:
- path: horus-ai-labs/gsm8k_sharegpt
split: train
template:
name: sharegpt
# eval_datasets:
# - path: horus-ai-labs/gsm8k_sharegpt
# split: test
# template:
# name: sharegpt
test_size: 0.1
streaming: false
distill:
type: logits
max_seq_length: 1024
sft_config:
output_dir: './results'
num_train_epochs: 2
per_device_train_batch_size: 8
per_device_eval_batch_size: 8
gradient_accumulation_steps: 8
eval_strategy: steps
eval_steps: 250
save_steps: 250
logging_steps: 1
learning_rate: 2.0e-5
weight_decay: 0.05
warmup_ratio: 0.1
lr_scheduler_type: 'cosine'
fp16: false
bf16: true
max_grad_norm: 1.0
remove_unused_columns: false
resume_from_checkpoint: null
temperature: 2.0
alpha: 0.5
Key Components:
- Student Model: Configured to use the smaller Qwen2-1.5B-Instruct model. The settings include optimizations like
flash_attn
andenable_liger_kernel
for efficiency. - Teacher Model: Uses the larger Qwen2-7B-Instruct model. This model’s outputs serve as the target for the student model during training.
- Data: Specifies the training dataset (here, GSM8K) and additional parameters such as the random seed and test size.
- Distillation Parameters: Under the
distill
section, the process is defined to use logits-based distillation. It includes training hyperparameters such as learning rate, batch sizes, number of epochs, evaluation and logging frequency, and more. The parameterstemperature
andalpha
control the softening of logits and the balance between distillation and the standard training loss.
Step 3: Launch the Distillation Process
After configuring the process, start the distillation by running the following command:
accelerate launch src/trainer.py --config config/gsm8k/logits_distill.yaml
This command leverages the accelerate
library to manage distributed training efficiently, following the configurations set in the YAML file.
By following these steps, you can effectively distill a large language model into a more compact version, leveraging the power of knowledge distillation with DistillFlow. Enjoy the process and the resulting efficiency gains!
Performance Benchmark and Comparison
To evaluate the effectiveness of our distilled model Qwen2-1.5B-Instruct-Distilled model relative to the original Qwen2-7B-Instruct model, we conducted benchmarks on two standard tasks: Hellaswag and GSM8K (with 5-shot prompting).
HellaSwag
Hellaswag is a benchmark designed to test commonsense reasoning and the model's ability to infer plausible continuations in everyday scenarios. The distilled model achieved 61.94 outperformed the original model score of 60.9 by 1.04, indicating that our distillation process preserved and slightly enhanced the model's reasoning capability on this task.
%20(1).png)
GSM8K (5-shot)
GSM8K is a math reasoning benchmark that evaluates the model's performance on complex, multi-step problems.On GSM8K (5-shot), the distilled model achieved a score of 46.17, marking a significant improvement over the original model’s score of 42.80. This result is particularly encouraging, as it shows that our distilled model can handle complex reasoning tasks more effectively.
%20(2).png)
How to deploy a Distilled LLM
Now, we will walk you through the process of deploying your distilled large language model (LLM) on a serverless GPU platform Inferless. We use vLLM for model inference, allowing you to scale your distilled model in production with minimal overhead.
Step 1: Create the Application File (app.py
)
Your app.py
file defines the inference logic by implementing three key functions: initialize, infer, and finalize.
initialize
: Loads the distilled model and sets up any required variables.infer
: Executes on every inference request. It processes the prompt, applies sampling parameters, and returns the generated output.finalize
: Cleans up resources and deallocates memory when the model is no longer needed.
Below is an improved version of the app.py
file with detailed inline comments:
from vllm import LLM
from vllm.sampling_params import SamplingParams
import inferless
from pydantic import BaseModel, Field
from typing import Optional
@inferless.request
class RequestObjects(BaseModel):
prompt: str = Field(default="Implement a function to check if a given number is a prime number.")
temperature: Optional[float] = 0.7
top_p: Optional[float] = 0.1
repetition_penalty: Optional[float] = 1.18
top_k: Optional[int] = 40
max_tokens: Optional[int] = 256
@inferless.response
class ResponseObjects(BaseModel):
generated_text: str = Field(default='Test output')
class InferlessPythonModel:
def initialize(self):
model_id = "Inferless/Qwen2-1.5B-Instruct-Distilled"
self.llm = LLM(model=model_id,enforce_eager=True)
def infer(self, request: RequestObjects) -> ResponseObjects:
sampling_params = SamplingParams(temperature=request.temperature,top_p=request.top_p,
repetition_penalty=request.repetition_penalty,
top_k=request.top_k,max_tokens=request.max_tokens
)
result = self.llm.generate(request.prompt, sampling_params)
result_output = [output.outputs[0].text for output in result]
generateObject = ResponseObjects(generated_text = result_output[0])
return generateObject
def finalize(self):
self.llm = None
Step 2: Define Your Custom Runtime Configuration
Inferless uses a custom runtime configuration file (inferless-runtime-config.yaml
) to set up your environment. This file specifies the CUDA version and the required Python packages.
Create a file named inferless-runtime-config.yaml
with the following content:
build:
cuda_version: "12.1.1"
python_packages:
- vllm==0.6.6.post1
- inferless==0.2.6
- pydantic==2.10.2
Step 3: Deploy the Model with Inferless CLI
Deploy your model effortlessly using the Inferless CLI. Follow these steps:
1. Initialize the Deployment Configuration : Run the command below to create a deployment configuration file. Replace Distill-LLM
with your preferred name.
inferless init --name Distill-LLM
2. Deploy to the Serverless GPU Platform : Use the following command to deploy your model. Specify the GPU type (e.g., A100
) and the custom runtime configuration file.
inferless deploy --gpu A100 --runtime inferless-runtime-config.yaml
Upon successful deployment, your distilled LLM is now deployed on the Inferless platform with cost-effective serverless GPU options, and you can begin sending inference requests.
Case Study
- Hugging Face's DistilBERT
Hugging Face developed DistilBERT, a distilled version of the BERT model, to address the need for more efficient natural language processing models. DistilBERT retains approximately 97% of BERT's language understanding capabilities while being 60% faster and 40% smaller. This reduction in size and increase in speed make it suitable for deployment on devices with limited computational power, such as smartphones and embedded systems.
- Amazon Alexa's Acoustic Models
Amazon applied knowledge distillation to improve the efficiency of acoustic models used in Alexa devices. By distilling knowledge from a large teacher model into a smaller student model, Amazon achieved significant reductions in model size without compromising performance. This allowed Alexa's speech recognition system to operate effectively on devices with constrained computational resources, enhancing user experience through faster response times.
- Improving Search Relevance on Roku Using CrossEncoders and LLMs
Roku have enhanced search relevance by combining bi-encoders with cross-encoder re-ranking. While bi-encoders efficiently retrieve candidates, they can miss the nuances of user intent, sometimes returning irrelevant results. To address this, the team fine-tuned shallow cross-encoders augmented by knowledge distillation from large language models (LLMs) to re-evaluate and rank search results more accurately. This hybrid approach reduced false positives, cut search abandonment by 4.5%, and boosted visit-to-stream rates by 2.4%, all while keeping latency and costs in check.
Challenges in Knowledge Distillation
While Knowledge Distillation holds the promise of making state-of-the-art language models more efficient and broadly available, it comes with its own set of challenges and considerations.
1. Teacher-Student Capacity Gap
A significant mismatch in capacity between the teacher and student models can hinder effective knowledge transfer. Large teachers often find solutions that are not within the solution space of smaller students, making it difficult for the student to mimic the teacher's behavior.
2. Teacher Model Quality
The performance of the student model is heavily dependent on the teacher model. If the teacher has limitations, such as biases or poor performance on specialized tasks, these shortcomings can be inherited by the student.
- Optimization Difficulties
Students often struggle to match the predictive distributions of teachers due to optimization challenges. Even when students have sufficient capacity, discrepancies between teacher and student outputs can persist, leading to suboptimal results.
- Hyperparameter Tuning
Effective distillation depends on carefully tuning hyperparameters, such as temperature settings for soft labels and loss functions. Identifying optimal values is complex and often requires extensive experimentation
5. Risk of Overfitting to Teacher Biases
Students may overfit to biases present in the teacher model or its training data, limiting their generalization ability and adaptability to new tasks or datasets.
6. Complexity of Knowledge Transfer
Transferring certain types of knowledge, such as intermediate feature representations or relational knowledge between data points, can be challenging. These forms of knowledge may require sophisticated algorithms for effective distillation.
7. Data Requirements
Knowledge distillation relies on substantial amounts of high-quality data used to train the teacher model. Limited or low-quality data can undermine the distillation process and reduce student model performance.
Conclusion
Knowledge distillation offers a powerful way to transfer the abilities of a large, high-performing language model to a smaller, more efficient student model. Through this process, we managed to distill Qwen2-7B-Instruct into Qwen2-1.5B-Instruct while not only preserving performance but, in some benchmarks, even surpassing the original model.
Beyond efficiency, the success of the distillation highlights a broader trend i.e the potential for advanced language capabilities to become more accessible, even under tighter computational and resource constraints. As LLM evolves, exploring and refining distillation workflows will likely remain integral to scaling and democratizing LLMs, with ongoing developments in model inference and applications.
Resources:
- https://klu.ai/glossary/knowledge-distillation-techniques
- https://labelbox.com/guides/knowledge-distillation/
- https://openaccess.thecvf.com/content_ICCV_2019/papers/Cho_On_the_Efficacy_of_Knowledge_Distillation_ICCV_2019_paper.pdf
- https://blog.roboflow.com/what-is-knowledge-distillation/
- https://openreview.net/forum?id=7J-fKoXiReA
- https://www.freecodecamp.org/news/knowledge-distillation-in-deep-learning-models/
- https://innodata.com/what-is-knowledge-distillation-in-ai/
- https://www.dcs.bbk.ac.uk/~sjmaybank/KD_Survey-arxiv.pdf
- https://www.sciencedirect.com/topics/computer-science/knowledge-distillation
- https://www.v7labs.com/blog/knowledge-distillation-guide
- https://jaketae.github.io/study/knowledge-distillation/
- https://www.larksuite.com/en_us/topics/ai-glossary/overview-of-knowledge-distillation-techniques
- https://www.ibm.com/topics/knowledge-distillation
- https://en.wikipedia.org/wiki/Knowledge_distillation
- https://openreview.net/forum?id=tcjMxpMJc95
- https://neptune.ai/blog/knowledge-distillation
- https://arxiv.org/html/2408.14678v1
- https://openaccess.thecvf.com/content/CVPR2022/papers/Beyer_Knowledge_Distillation_A_Good_Teacher_Is_Patient_and_Consistent_CVPR_2022_paper.pdf