DeepSpeed MII vs. Triton Inference Server: Which Inference Solution is Right for Your LLMs?
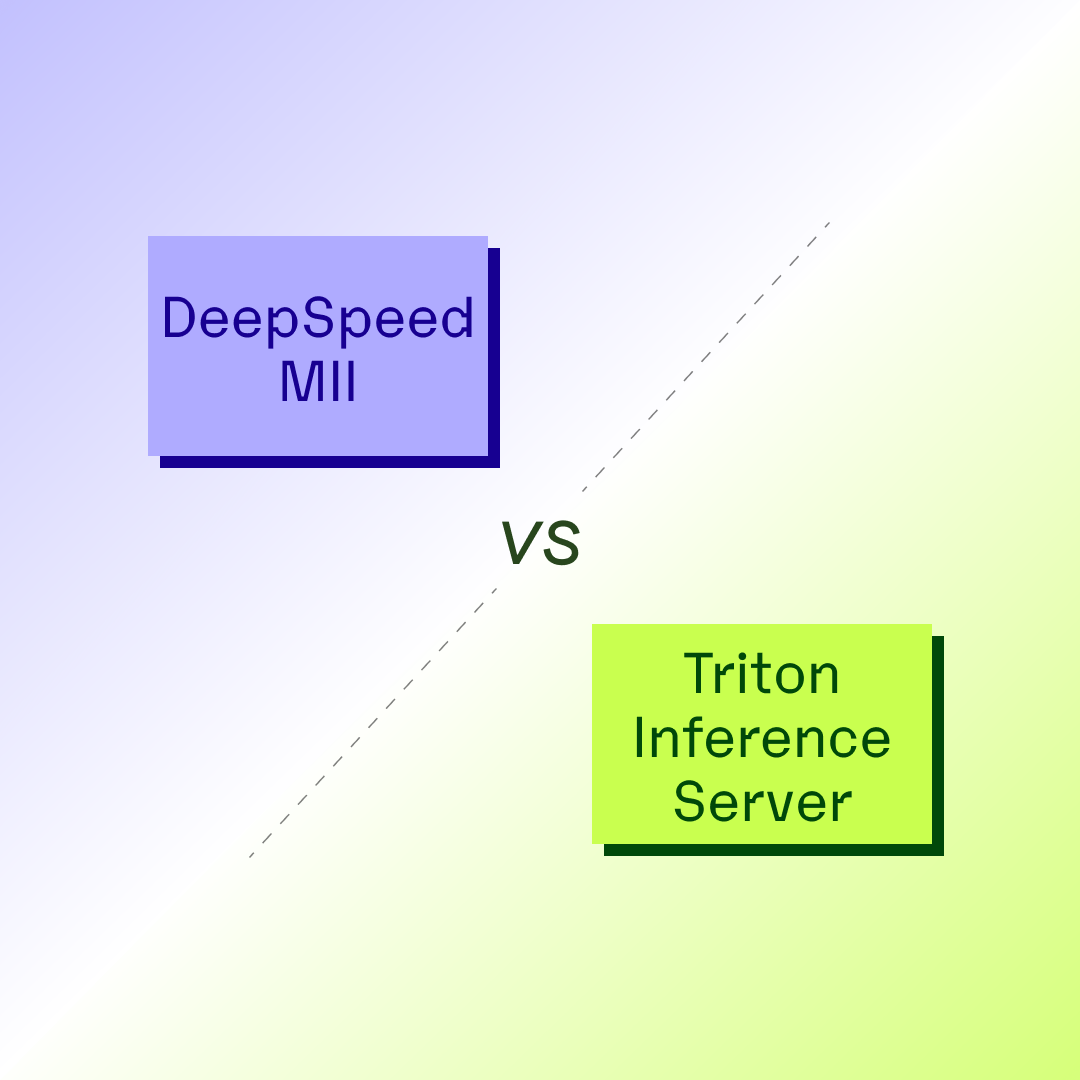
Introduction
This blog explores two inference libraries: DeepSpeed MII and Triton Inference Server. Both are designed to optimize the deployment and execution of LLMs, focusing on speed and efficiency.
DeepSpeed MII, an open-source Python library developed by Microsoft, aims to make powerful model inference accessible, emphasizing high throughput, low latency, and cost efficiency.
Triton Inference Server, developed by NVIDIA, is an open-source inference server that streamlines the deployment and management of AI models across diverse environments, supporting multiple frameworks and optimizing performance through its features.
Performance Metrics
DeepSpeed MII and Triton Inference Server are popular solutions for deploying large language models (LLMs), renowned for their efficiency and performance. We will compare them based on latency, throughput, and time to first token (TTFT):
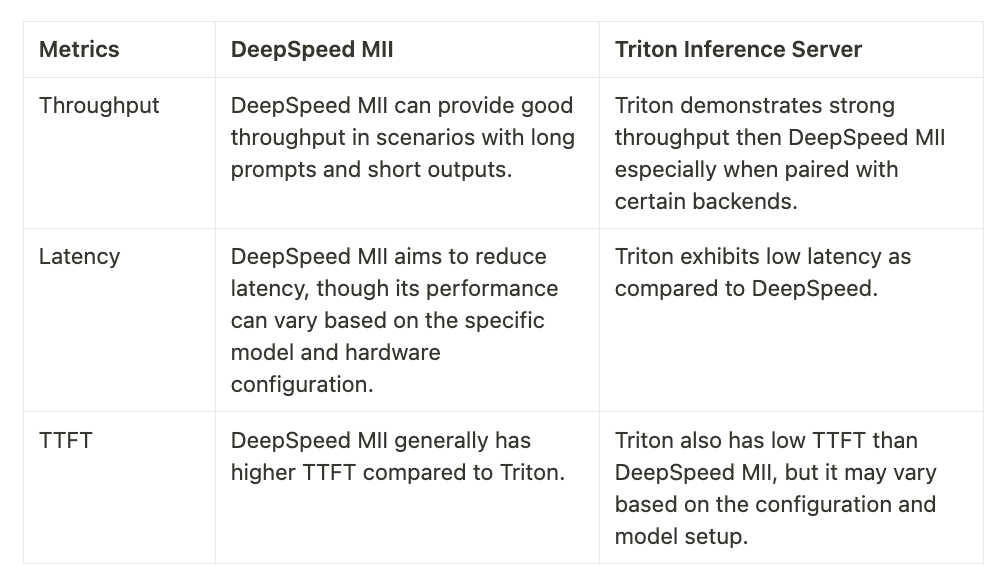
Features
Both DeepSpeed MII and Triton Inference Server offer robust capabilities for serving large language models efficiently. Below is a detailed comparison of their features:
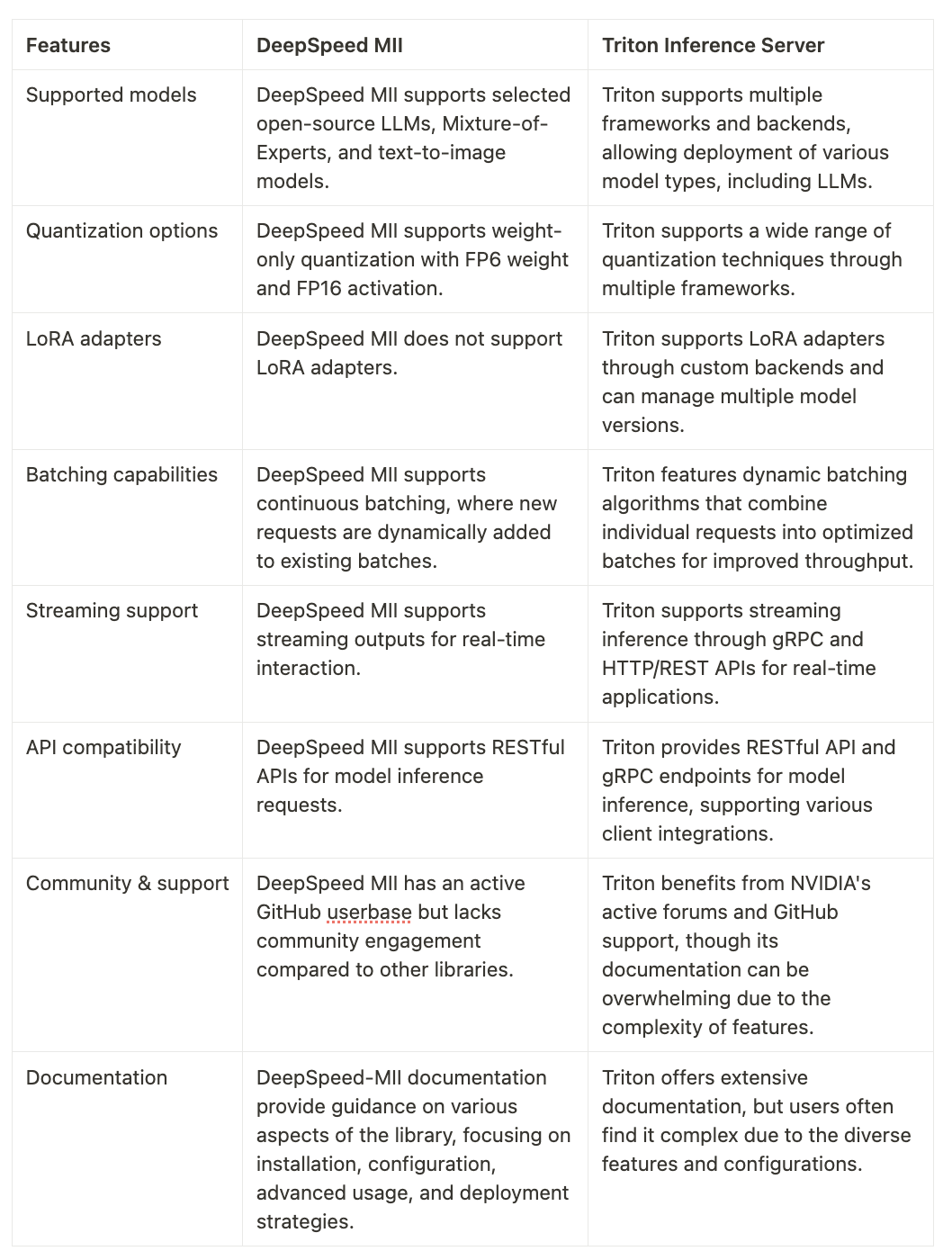
Ease of Use
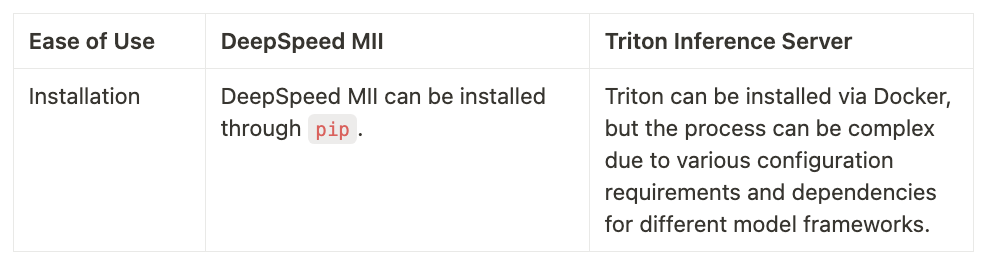
Scalability
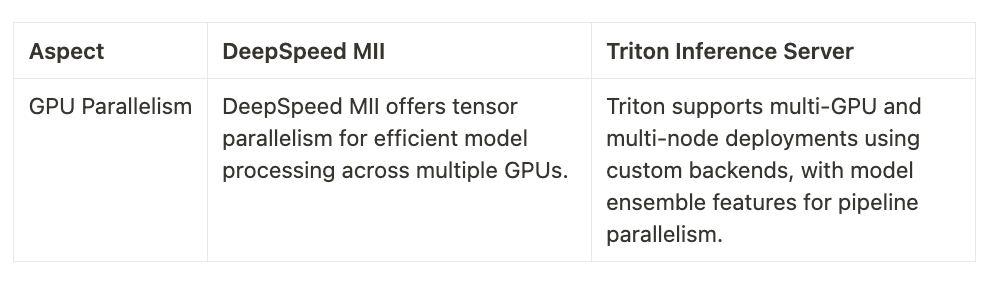
Integration
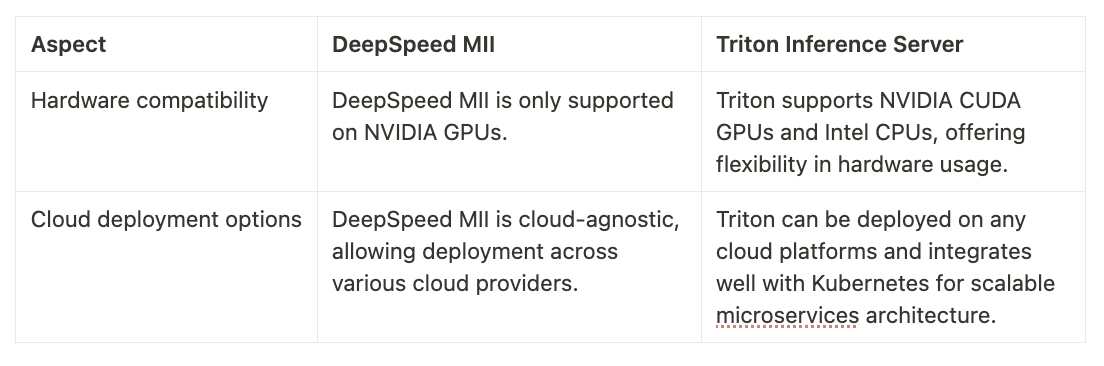
Conclusion
Both DeepSpeed MII and Triton Inference Server offer powerful solutions for serving large language models (LLMs), each with unique strengths tailored to different deployment needs. DeepSpeed MII excels in scenarios involving long prompts and short outputs, with a focus on low-cost weight-only quantization. On the other hand, Triton excels in providing a robust inference server environment that supports multiple frameworks and offers advanced features such as model ensemble capabilities for pipeline parallelism.
Ultimately, the choice between DeepSpeed MII and Triton Inference Server will depend on specific project requirements, including performance metrics, ease of use, and existing infrastructure. As the demand for efficient LLM serving continues to grow, both libraries are poised to play critical roles in advancing AI applications across various industries.
Resources
- https://github.com/microsoft/DeepSpeed-MII
- https://github.com/triton-inference-server/server
- https://deepspeed-mii.readthedocs.io/en/latest/
- https://docs.nvidia.com/deeplearning/triton-inference-server/user-guide/docs/index.html