How SpoofSense scaled their AI Inference with Inferless Dynamic Batching & Autoscaling
Jun 27, 2024
When it comes to real-time AI inference, performance can make or break the usage of your product. For SpoofSense, pioneering a facial AI spoofing detection product used by renowned companies like Ongrid, Bureau the challenge wasn't just building cutting-edge AI—it was deploying it at scale with blazing speed.
This is a case-study of how Inferless helped SpoofSense overcome critical performance hurdles and achieve high QPS and low latency with our dynamic batching and autoscaling features.
The Challenge
Imagine this scenario: Your AI model is so accurate and in-demand that your infrastructure becomes your biggest bottleneck. This was the reality for SpoofSense. As Kartikeya, Founder of SpoofSense, explains:
"We suddenly got a lot of customers that wanted to use our models at a very high QPS as well as they wanted a very low latency. And it was really difficult for us to, in a short time, build an inference platform, build our inference infrastructure at our end."
The performance requirements were staggering:
- Customer demand: Up to 200 queries per second (QPS)
- Required latency: P95 under 2-3 seconds
- Available DevOps expertise: Limited
SpoofSense found themselves at a critical juncture—their product was cutting-edge, but their ability to serve it efficiently was holding them back.
The Nvidia Triton Inference Server Challenges: When DIY Falls Short
Before discovering Inferless, SpoofSense attempted to tackle the challenge head-on with NVIDIA's Triton Inference Server themselves. Kartikeya recounts their experience:
"We tried deploying the Nvidia Triton server on our own, but we could not get it to scale across multiple machines and have a consistent experience while autoscaling that we require for our customers."
Despite Nvidia Triton's powerful features, the complexities of deployment, scaling, and optimization proved to be a significant hurdle for a team focused on AI innovation rather than infrastructure management.
Solution:
With Inferless, SpoofSense was able to deploy their AI models effortlessly, achieving impressive auto scaling and maintaining low latencies crucial for real-time image analysis. Inferless's platform allowed SpoofSense to handle up to 200 QPS with P95 latencies under three seconds, a benchmark that was previously unattainable.
"Inferless not only simplified our deployment process but also enhanced our model's performance across varying loads using dynamic batching," Kartikeya explained. The seamless integration with Google Cloud Buckets via the console made model deployment a breeze, allowing instant updates and live deployments as soon as models were trained.
The Impact
Switching to Inferless has also led to substantial cost savings of up to 60% for SpoofSense as compared to managing on-demand GPU clusters themselves. These savings stem from reduced needs for specialized DevOps personnel, minimized underutilized GPU time, and the ability to downscale resources automatically during low traffic periods. The user-friendly console, real-time analytics, and the infrastructure's reliability ensure that SpoofSense can focus on their core mission of advancing AI in image authenticity without the overhead of managing complex infrastructure.
The Secret Sauce: Why Inferless Delivered Where Others Couldn't
Inferless's success with SpoofSense wasn't just about raw performance. It was about understanding the unique challenges faced by AI-first companies:
- Developer Velocity:"If you have a model ready, you don't have to sort of wait for your DevOps engineer or your MLOps engineer to create the infra and then serve your model." - Kartikeya
- Performance:As their performance needs evolved with latency & autoscaling, Inferless cold-starts of less than 10 seconds helped them scaled effortlessly & consistently providing the same performance whether its 10 users OR 1000 users.
- Performance Insights: Real-time analytics on API calls, traffic scaling, and latency helped SpoofSense continually optimize their models and understand usage patterns.
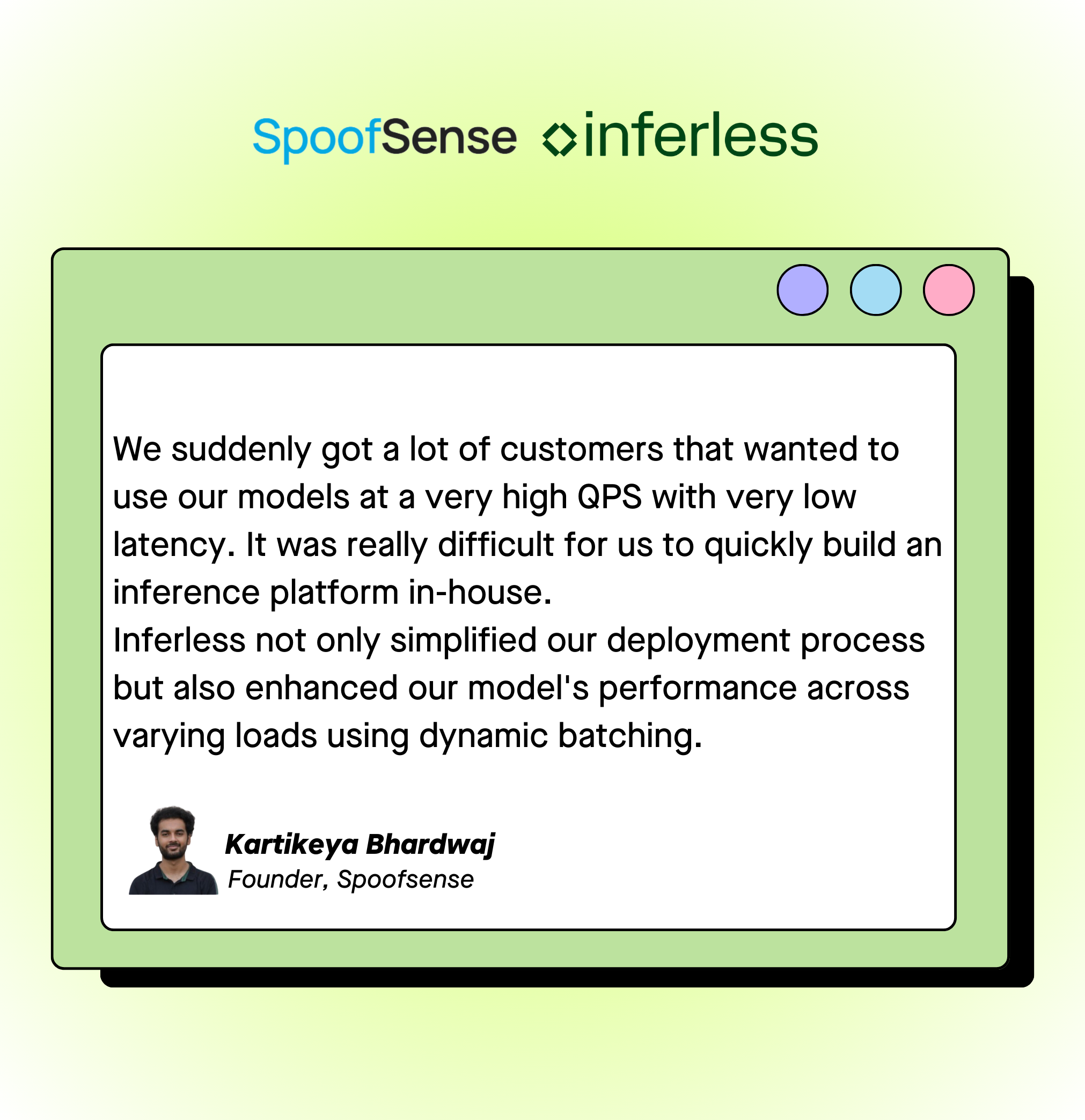